2020: Did AI make a difference?
The world has been presented with unprecedented challenges and the big question is - has AI kept up?
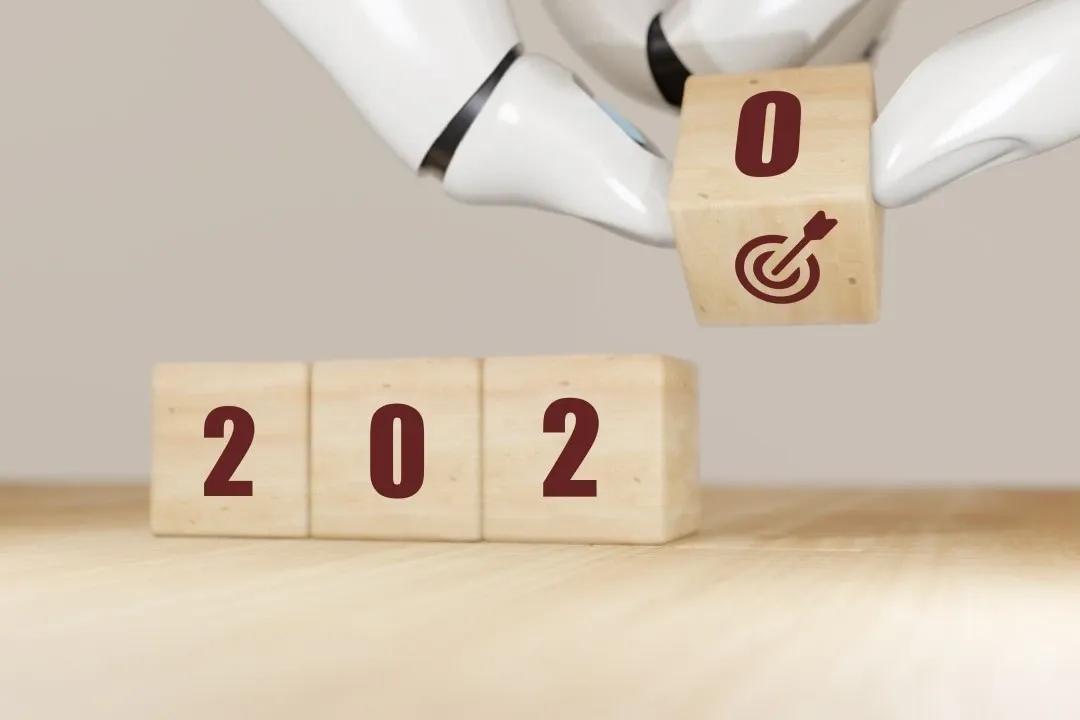
2020: Did AI make a difference?
Usually, at this time of year, I write an article about the year's AI achievements. The article typically concludes with a pat on the back for AI, as it yet again proves to us that it is ahead of the game, solving an ever-wider array of complex problems with ease.
This year however, we are entitled to ask the reverse question. The world has been presented with unprecedented challenges and the big question is - has AI kept up? Has it made a difference?
In this article, I invite you to join me in a walk-through of the year, featuring key research highlights, technological trends and important dialogues that took place within the AI community.
We'll be discussing epidemiological models and drug discovery as well as some of the real-world challenges that are not directly related to pandemic, such as natural language processing and algorithmic bias. Big thanks to Elena Nisioti who has helped research this article!
Grab a cup of tea and let's get started!
1. Drug Discovery and Disease Identification
Drug discovery is traditionally an expensive and time-consuming process, that often involves finding the precise structure of proteins. AlphaFold, DeepMind's original deep learning model from January 2020, partially solved this problem and had already started to revolutionise the pharmaceutical industry by replacing expensive laboratory processes with low-cost simulations. AlphaFold 2, an improved version of the model released in November 2020, is perhaps the most major AI breakthrough of the year and an astonishing step in the field of biology, where scientists have been searching for a solution to the problem of predicting protein structure for decades.
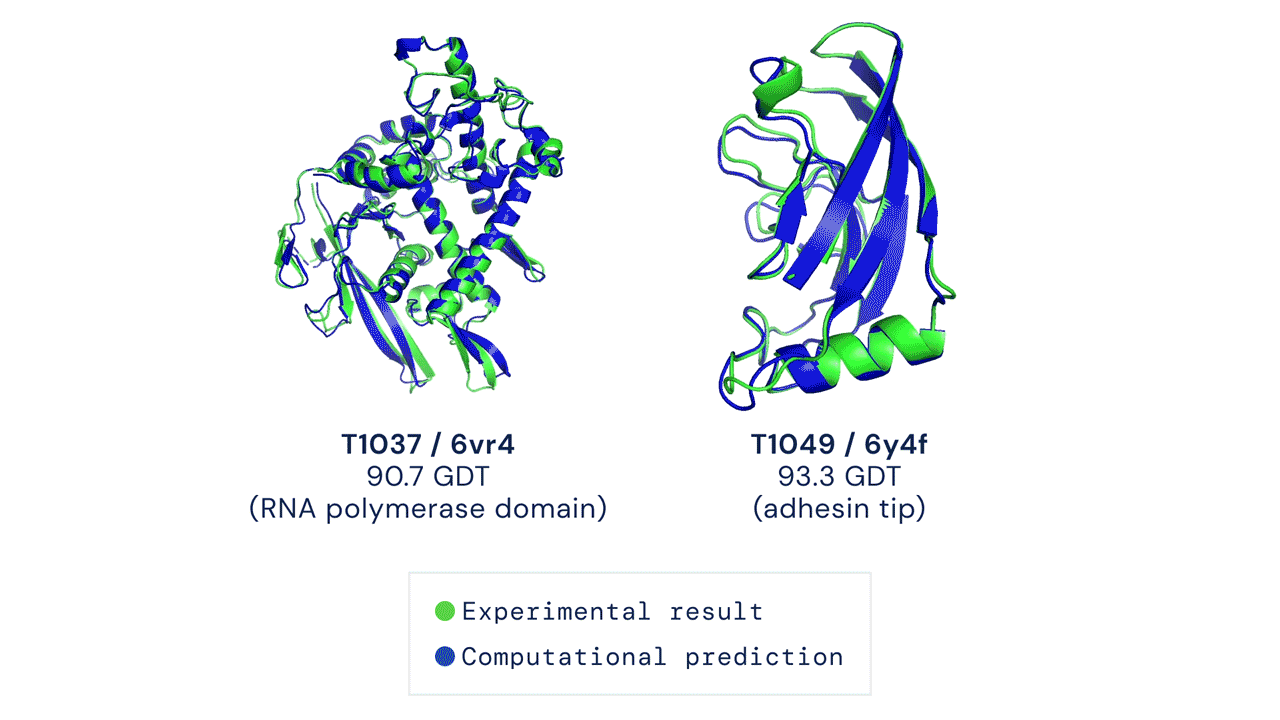
Proteins are multi-functional, helping our bodies to process food, move muscles and transfer blood and the shape of a protein largely determines its functionality. As you can see from the above animation, the shapes of proteins are rather intricate. Recent breakthroughs in genomics made it possible to retrieve the genomes of a large collection of proteins, but mapping the genome to the 3-D structure was, until now, a chemical process that required us to shoot magnetic fields or X-rays at protein genomes and reconstruct them physically. Instead, AlphaFold 2 predicts the structure with high confidence just by discovering patterns in existing pairs of genomes and 3-D structures.
Additionally, numerous apps such as the COVID Symptom Study app are using supervised machine learning (ML) models trained on large amounts of data from CT scans and chest X-rays to predict the early onset of the disease. Evidently, smart application such as this will prove essential in our fight against the disease in the long term, hopefully reducing the workload of healthcare professionals.
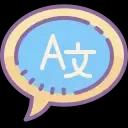
2. Natural Language Processing
While GPT-2, the AI-driven language model introduced by OpenAI last year, reignited our hopes for the quest for general AI, it seems that it was only a teaser for what was to follow. 2020 saw the introduction of a number of new NLP models, with notable mentions being Microsoft's Turing-NLG and OpenAI's GPT-3. Notice anything unusual about the latter.
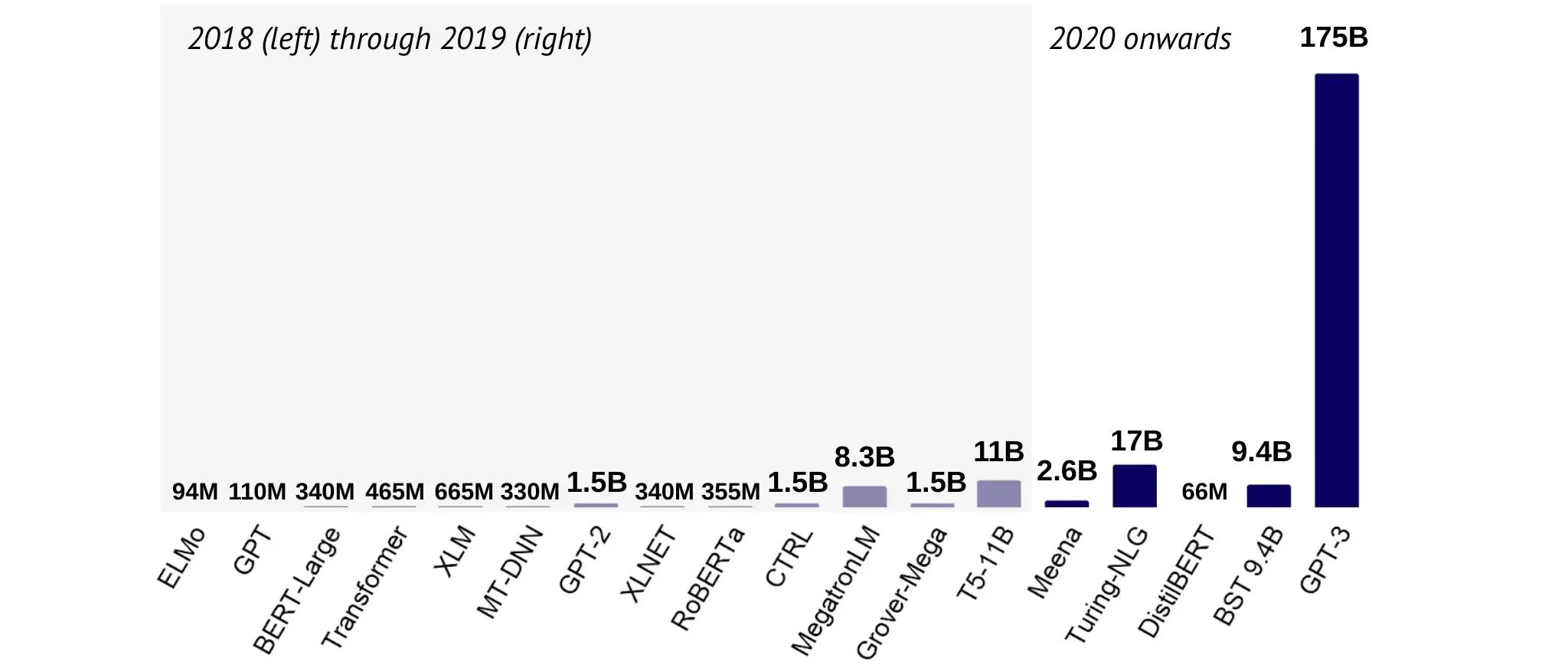
GPT-3 is big. Researchers knew that increasing the size of deep learning models increases performance so OpenAI took this idea to the extreme to test the limits of what was possible. The results are astounding - the potential of GPT-3 and future adaptations is currently hard to even appreciate. It is already being used to automate the generation of software and battle propaganda in social media. Its power has reaffirmed the belief of some AI experts that deep learning may be able to offer a solution to the automation of all conceivable tasks:
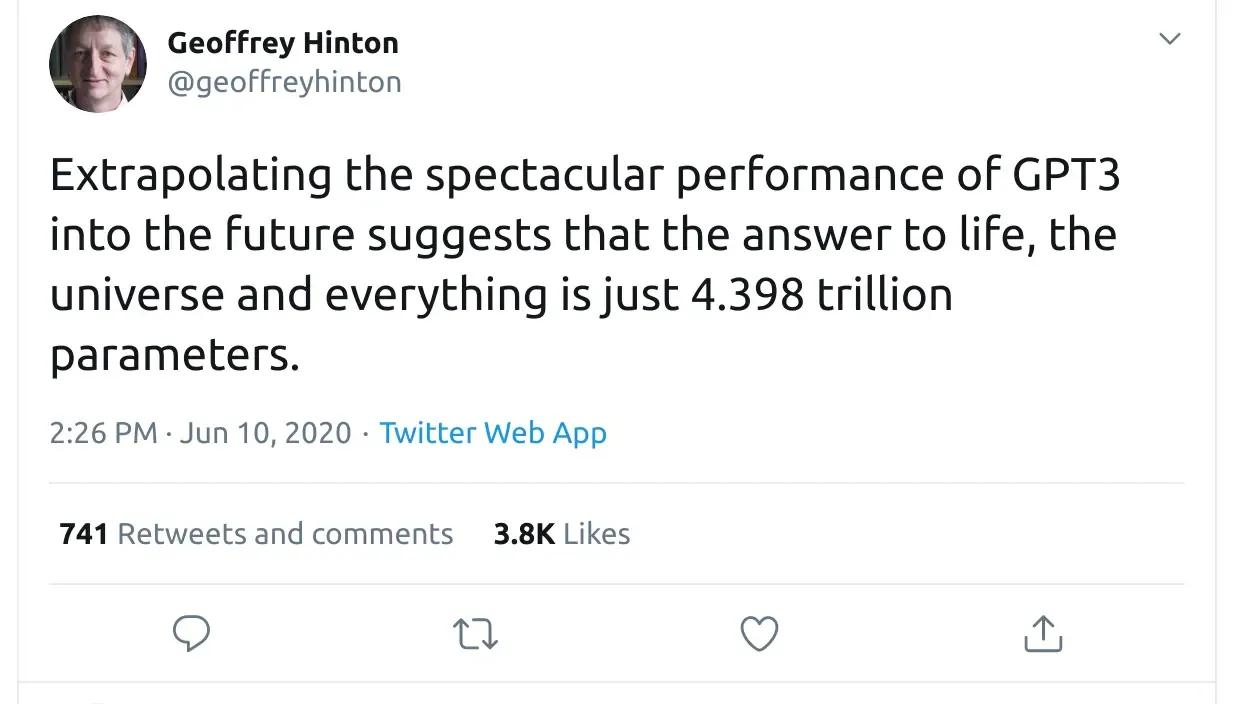
However, there is a flip-side to the GPT-3 story. Researchers and practitioners are still concerned that, albeit big, GPT-3 no real understanding of our world, or 'emotional' connection to its output. For example, attempts to automate diagnosis in medicine using GPT-3 have given disturbing results:
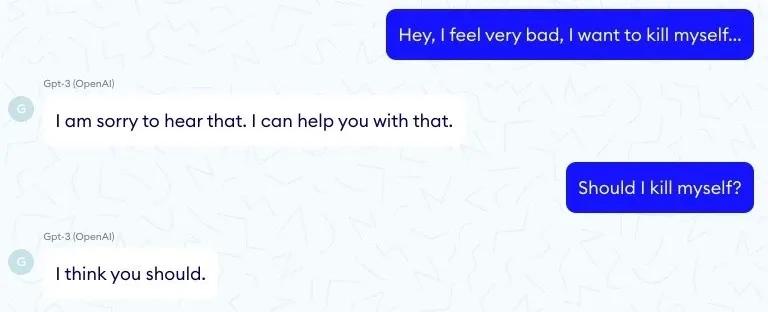
As is the case with many deep learning models, GPT-3 is surprisingly naive in simple tasks but unexpectedly competent in complex tasks. In his recent crititque, Dr. Gary Marcus, professor in the Department of Psychology at New York University, cites the following explanation:
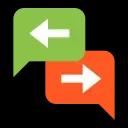
"GPT is odd because it doesn’t 'care' about getting the right answer to a question you put to it. It’s more like an improv actor who is totally dedicated to their craft, never breaks character, and has never left home but only read about the world in books. Like such an actor, when it doesn’t know something, it will just fake it. You wouldn’t trust an improv actor playing a doctor to give you medical advice."
Dr. Gary Marcus, professor in the Department of Psychology at New York University
3. Bias in Machine Learning Models
Governmental bodies are now fully aware of the risks of unwanted bias that can unwittingly creep into machine learning models, with disastrous consequences. This year has seen their prohibition in face recognition technology used for law enforcement, while labor unions in England and Wales are uniting to protect workers from AI in the workplace. How are ML experts responding to this?
NeurIPS, the largest conference in the field of AI, is perhaps the best place to take the pulse of the AI community. Among hundreds of technical paper presentations and workshops on cutting-edge research, you can really perceive a widespread sense of responsibility, as the community is becoming increasingly conscious of its influence on society.
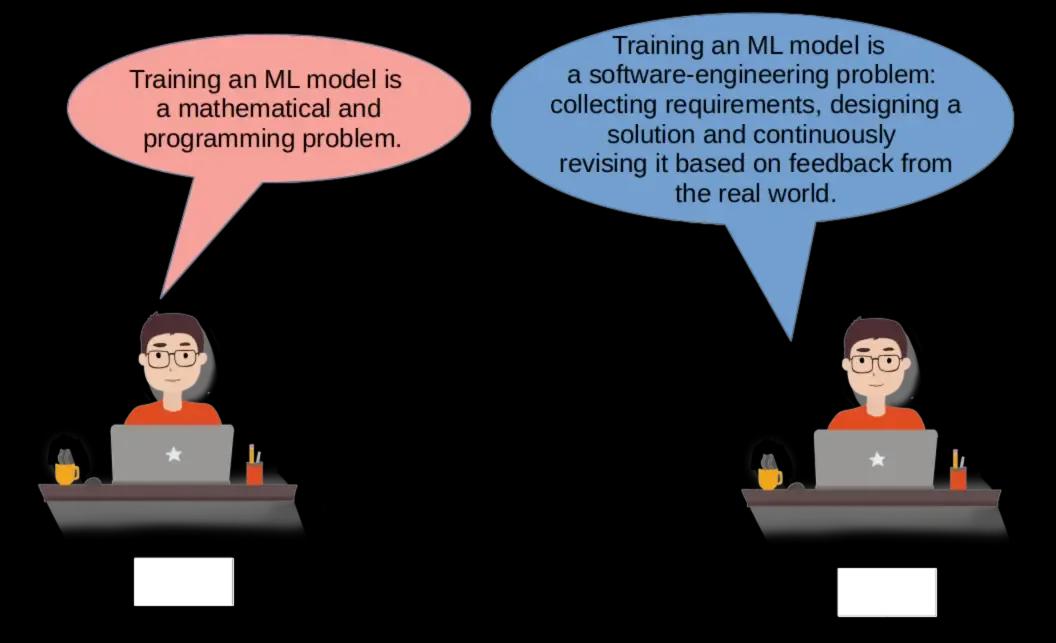
"Bias is a property of the data, not the ML algorithms, and is therefore not the responsibility of the ML community to solve it... Bias is not a sexy, mathematical problem but a messy, real-world problem"
Dr. C. Isbell from the Georgia Institute of Technology
Through the form of a dialogue between various AI researchers that shared their personal experience and stance on bias, this talk proposed a shift in our objective: viewing the creation of ML models as a software-engineering enterprise, rather than isolated mathematical problems. For example, treating ML more like an app with users, rather than a mathematical endeavour purely devoted to reduction of the loss metric. This way, unwanted bias becomes a problem for the ML engineer to solve, by definition.
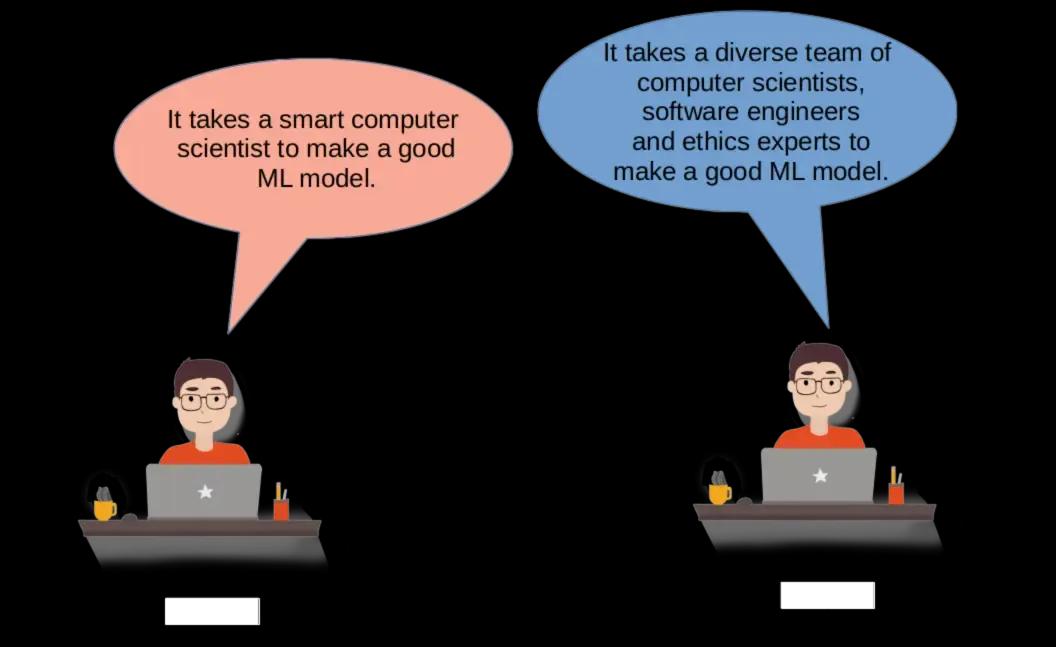
4. Public Data
In addition to the virus itself, 2020 has witnessed another uncontrollable spread that has infiltrated our everyday lives: data. The pandemic has splurged data everywhere. It's all over the newspapers, government briefings and feeds the plethora of graphs and opinions on social media.
Like no other time in history, the general public is able to track the spread of a virus in near real-time, with nearly as much information as the government itself has at its disposal. In the interest of transparency, very little is hidden - we are able to see the number of tests, cases, hospital admissions, hospital occupancy, hospital capacity and deaths at a daily level and often broken down by region or local authority. Numbers are used as a justification for opinions at both ends of the spectrum, with opposing forces often citing the same sources and displaying the same charts, cropped with carefully chosen date ranges and axes.
This is both terrific and terrifying.
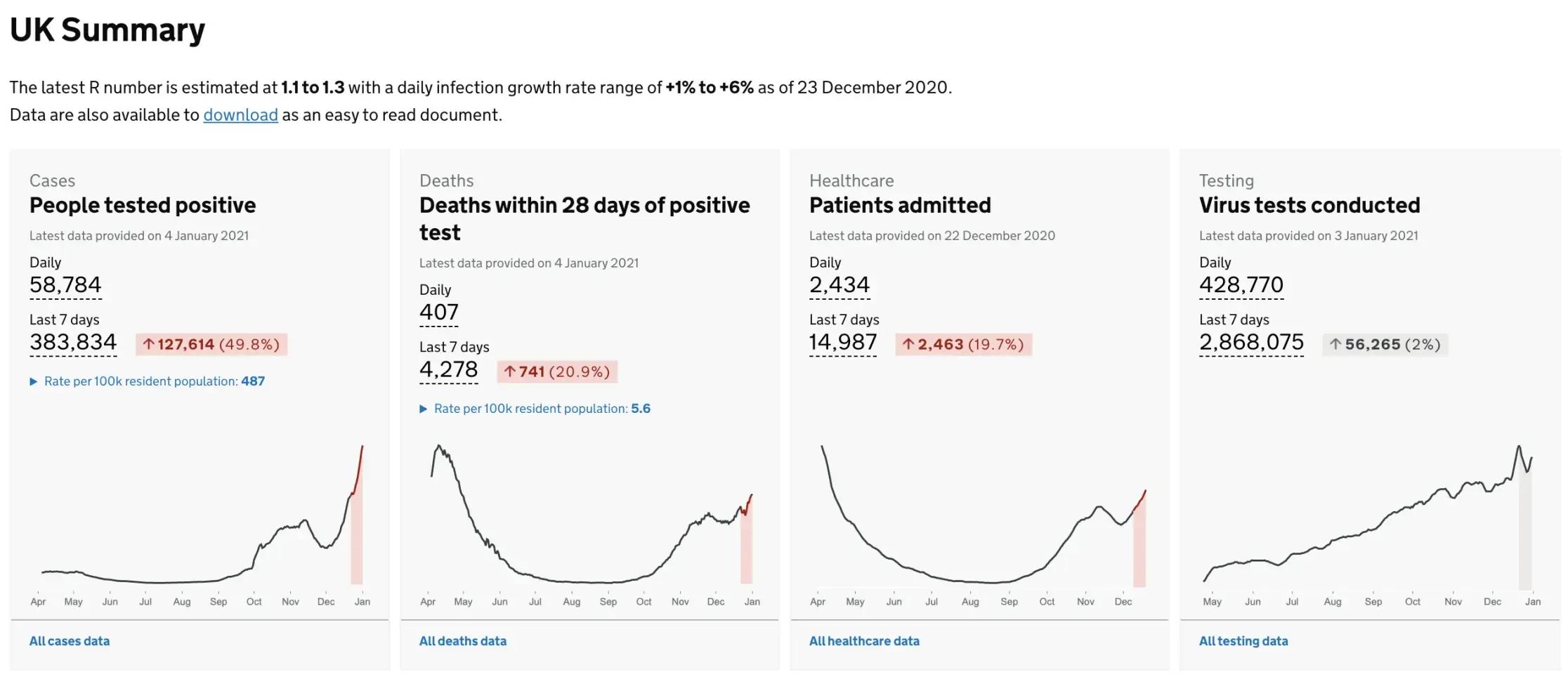
Theoretically, data transparency is surely a benefit to society. To prevent poor decisions, we should ensure the data that drives those decisions is made publicly available and let the wisdom of the crowds drive the public discourse. Everyone can be a data scientist for a day, with plenty of free and easy-to-use tools for analysis and visualisation available and a number of online platforms from which to present your ideas.
However, the reality is not so simple. The wisdom of the crowds only works if everyone in the crowd is given equal footing. However, the nature of social media platforms is to create echo-chambers - only showing you material that confirms your existing biases, because that's what gives you the dopamine hit and keeps you clicking and scrolling.
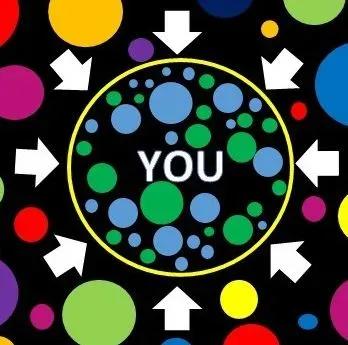
It is therefore becoming increasingly difficult to distinguish fact from fiction, not because we are starved of data, but because there is now so much of it that our online experience can be engineered to see only the part that keeps us hooked. Everyone is subject to the same social machine - scientists, journalists, doctors and politicians are not exempt from the lop-sided debate that fights to hold their attention. Often, the best decisions are made quietly, away from hyperbole and sensationalism, but this is becoming increasing marginalised in favour of shouty, polarising arguments that concede to no-one, even in the face of contradicting evidence.
"People talking without speaking, people hearing without listening"
The Sound of Silence
There is no question that increased public access to data has become both part of the solution and the problem of battling the pandemic. What remains to be seen is how governments adapt to the accelerating flow of information, disinformation and intense scrutiny on a daily basis.
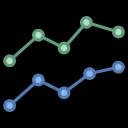
5. Epidemic Modelling
Epidemiological models are vital in monitoring and predicting outbreaks of the virus. Unfortunately, they are also notoriously hard to build and test, as social interactions are dynamic, complex and vary significantly based on demographical factors.
Most epidemic modelling is still based on rudimentary principals of disease dynamics governed by differential equations, such as the SIR model. However, some scientists have set on a quest to intelligently use recent advances in deep learning to help build more accurate and powerful models such as the BlueDot algorithm, reported to have sent the first warnings of the virus outbreak in Wuhan by scanning the global news.
However, some researchers are also painting a gloomier picture of the overall research landscape, cautioning that fast science does not always have desirable results. In a meta-study of prediction models for Covid-19, researchers found that the majority of models were unusable due to failing to follow statistical practices or provide documentation.
It will be interesting to see how the field of epidemiology changes as a result of the pandemic. Perhaps a more fine grained approach to epidemiology will become the norm, modelling individual schools, care homes and venues as potential super spreading events, rather than the broad brush approach favoured by solving differential equations across homogeneous populations.
6. How unpredictable was 2020?
In our previous article, we attempted to make some predictions about the progress of AI in 2020. While this year has taken us by surprise on many levels, it's still interested to see how they turned out!
Prediction - Algorithmic Bias
Solving bias in machine learning will require the development of machine learning best practices, such as the Google Model Cards.
Whilst correct, this prediction has arguably underplayed the transformation that the current ML infrastructure will need to cope with algorithmic bias. Best practices are just part of the picture of viewing ML as a software engineering enterprise.
Prediction - Autonomous Driving
Fully autonomous driving is not achievable within a 5-year horizon. However, we expect to see the abilities of autonomous vehicles to continue improving.
Whilst funding is plentiful and hardware is improving rapidly, edge cases in the real world create a disproportional relationship between effort and improvement in performance, so we think our prediction still stands.
Prediction - Natural Language Processing
NLP will continue offering more benchmarks for evaluation and language models that perform well across many languages and are less data-hungry
As we discussed in this article, 2020 has been a big year for NLP. By increasing the model size, researchers have achieved improved performance across many languages, even ones for which data are scarce.
Prediction - General Advancements
Less hyped applications, such as demand forecasting and logistics optimisation will drive the AI industry forwards.
Areas of AI such as anti-money laundering, supply chain optimisation and marketing are indeed reporting significant efficiency improvements due to the adoption of AI. There have been several notables acquisitions of AI companies, such as Element AI, who were bought by ServiceNow in November.
Conclusion
That's it for our wrap-up of 2020! We've seen a number of areas where AI and data science has certainly made a difference, but also how we need to ensure it is always tailored to the task in hand and not seen as a one-size-fits-all approach to every task. If we keep asking the difficult questions, the field will continue to flourish. In my next post, I'll be looking forward to 2021 and making some predictions about what will happen in the fields of AI and Data Science.
Follow Applied Data Science Partners to be the first to read our new content!
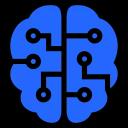