Definitive Guide to Auditing Model Bias Guide 2025
Discover the importance of auditing model bias in AI, learn about various bias types and how to mitigate.
Understanding Model Bias
Artificial Intelligence (AI) is now a cornerstone of many industries, including healthcare, finance, and education. As AI systems increasingly influence important decisions, ensuring fairness and unbiased outcomes is critical. Model bias occurs when an AI system consistently favours certain groups or outcomes due to factors like training data, algorithm design, or optimisation objectives. For example, a facial recognition system trained mostly on a single ethnic group may fail to recognise faces from other groups, illustrating data bias. Addressing model bias is essential for creating fair and ethical AI systems.
Why This Guide?
In this guide, we explore the importance of auditing model bias in AI, focusing on fairness, legal compliance, public trust, accuracy, and ethical responsibility. Ensuring fairness in AI prevents discrimination in critical areas like hiring and lending. Legal and regulatory requirements necessitate audits to avoid penalties. Public trust in AI technology hinges on unbiased systems. Addressing bias improves model accuracy and aligns with our ethical duty to use AI for societal betterment.
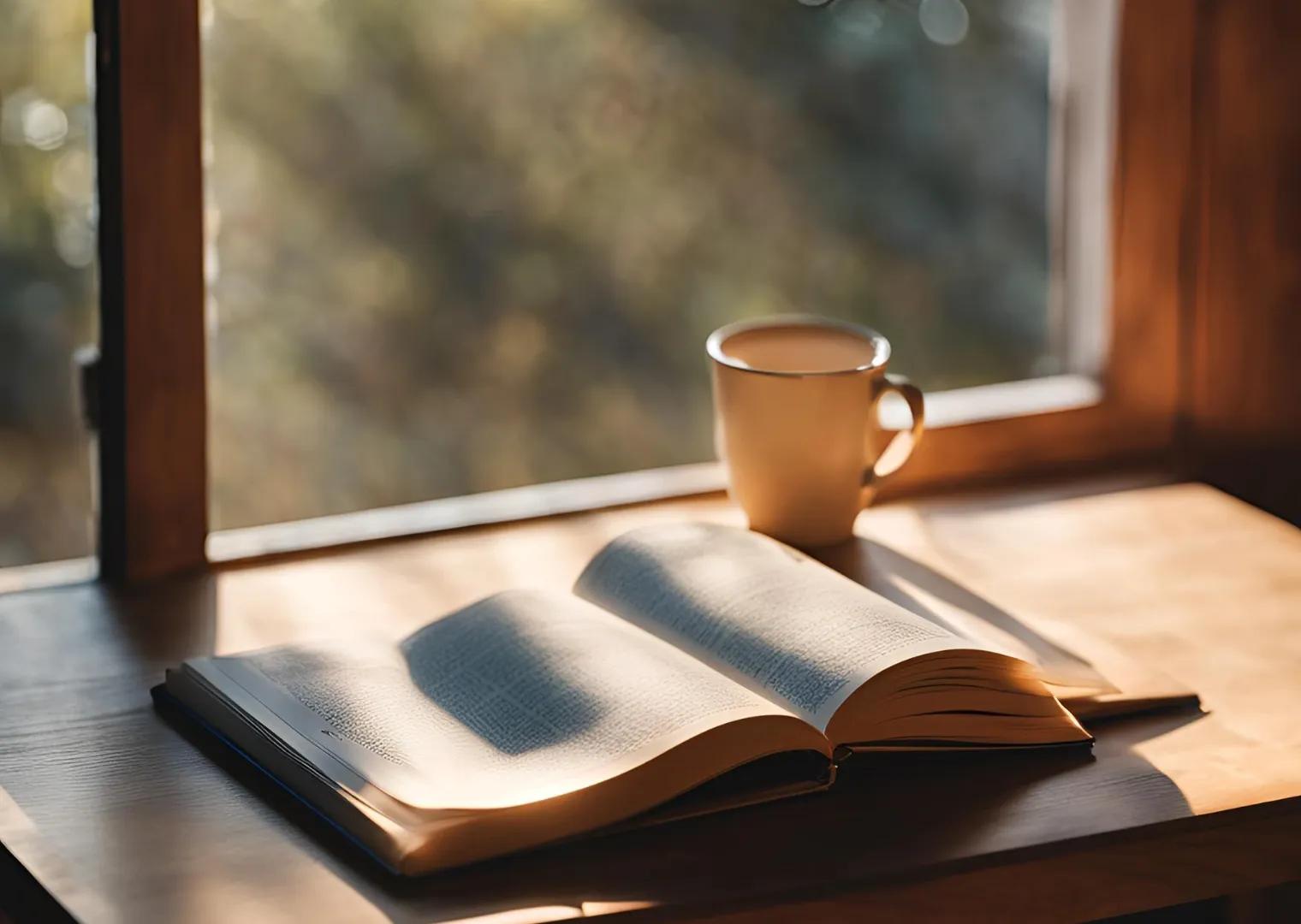
Chapters Overview:
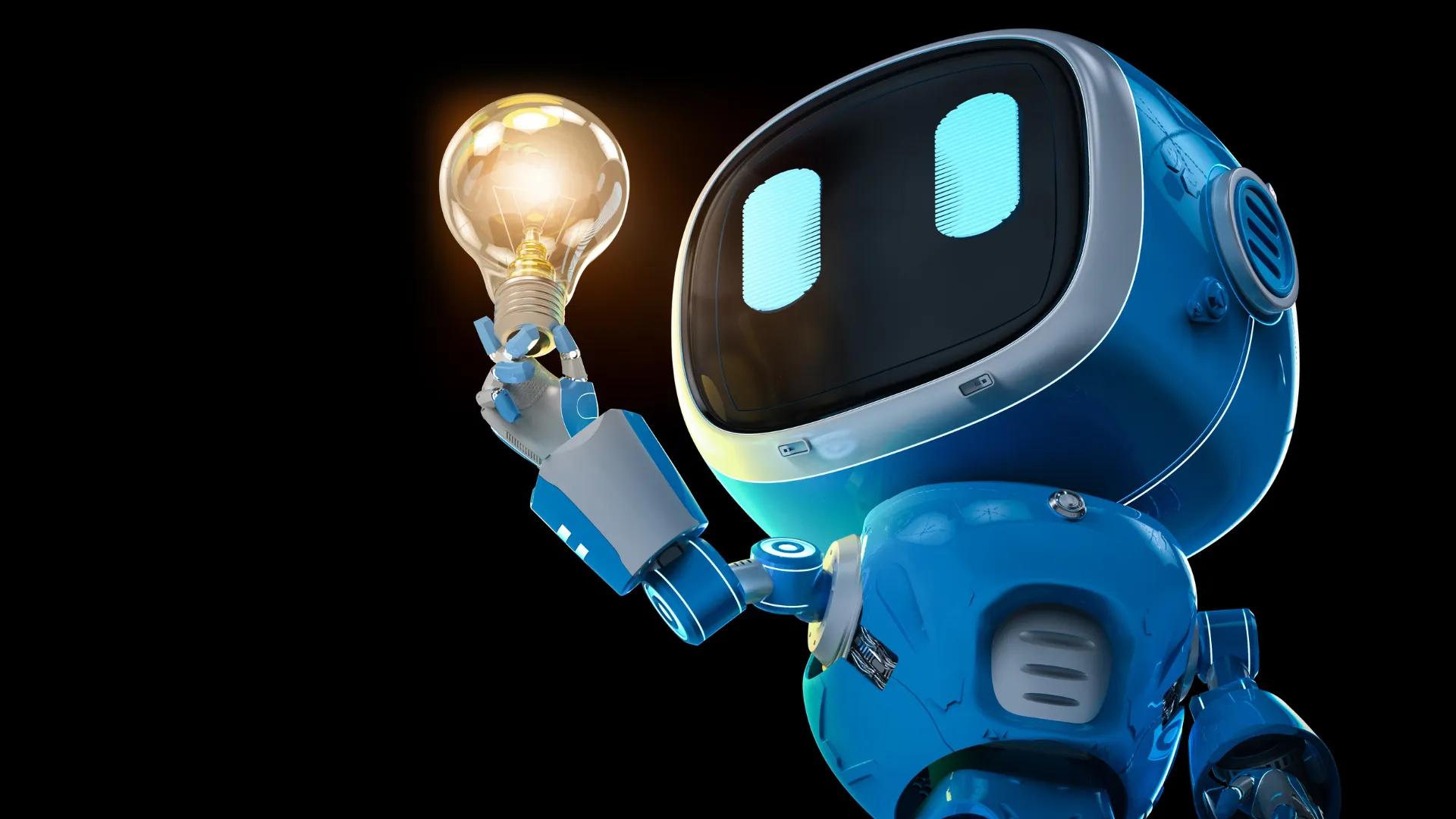
1. Understanding Bias in AI
As AI systems continue to make significant impacts across various sectors, it is imperative to understand and address the biases that can be inherent in these systems.
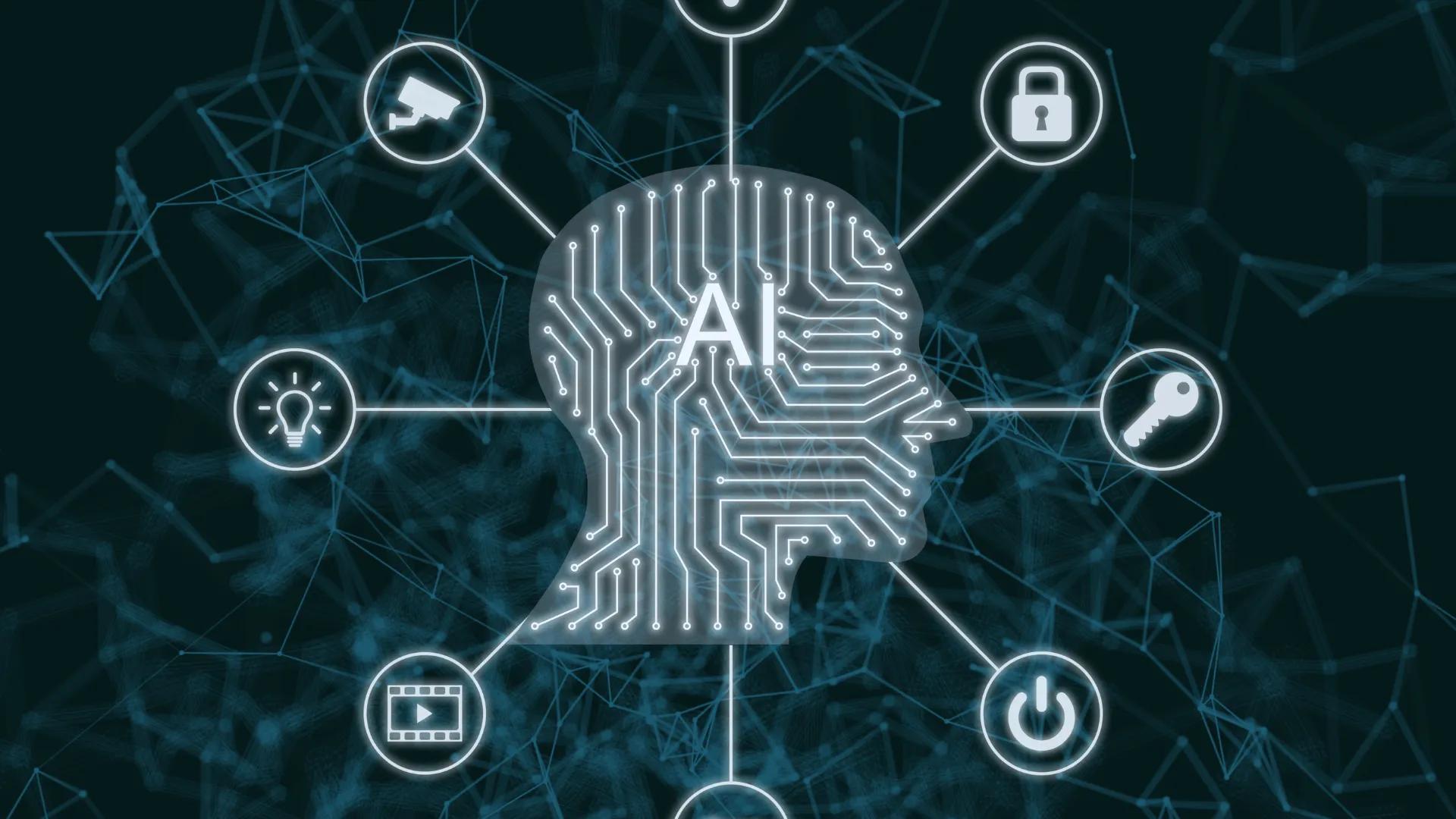
2. Types of Bias in AI
Explore the different types of bias in AI, including data bias, label bias, selection bias, measurement bias, algorithmic bias, confirmation bias, and automation bias.
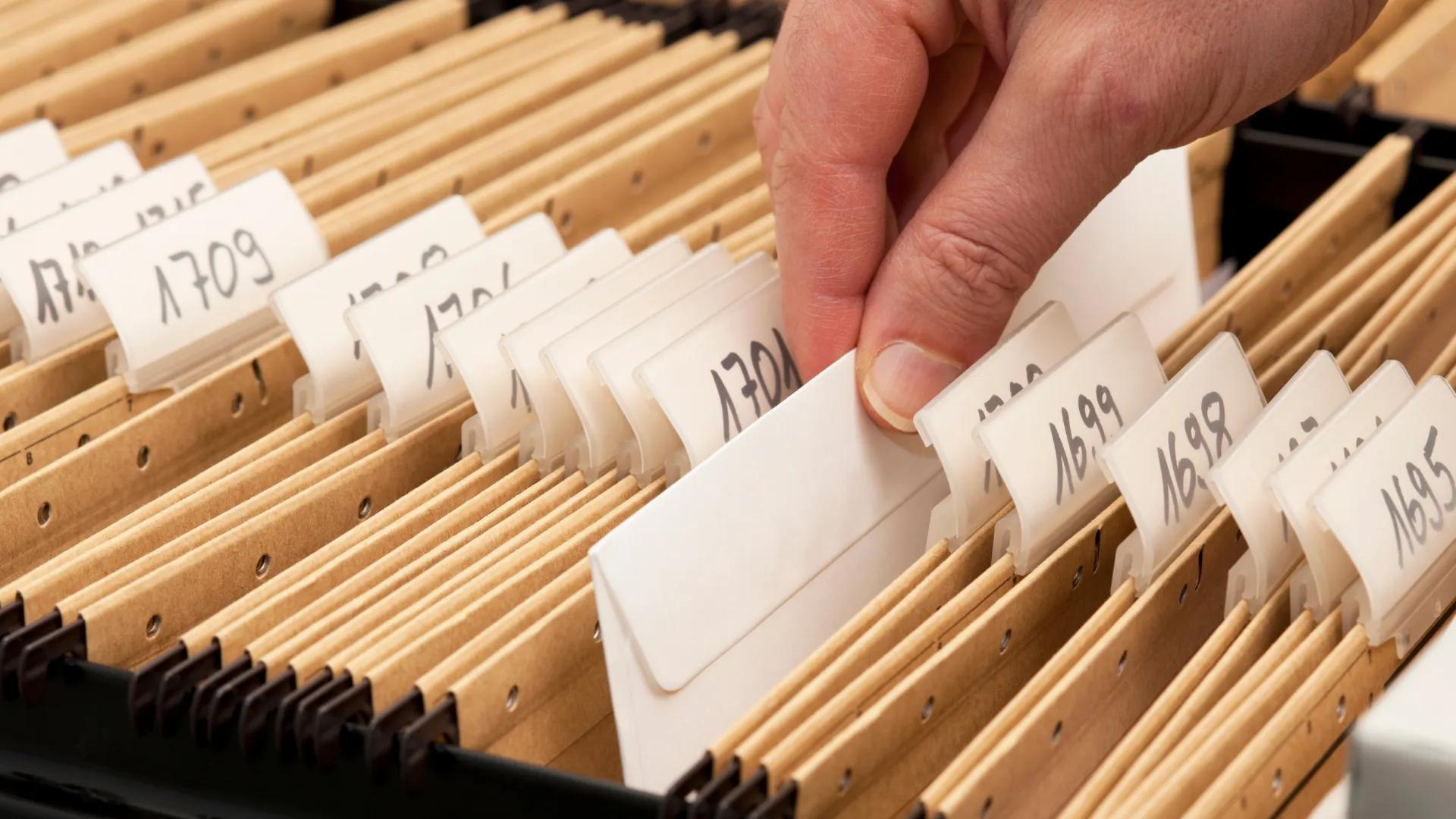
3. Sources of Bias
Understand the various sources of bias in AI, such as historical data, human prejudices, and data collection methods.
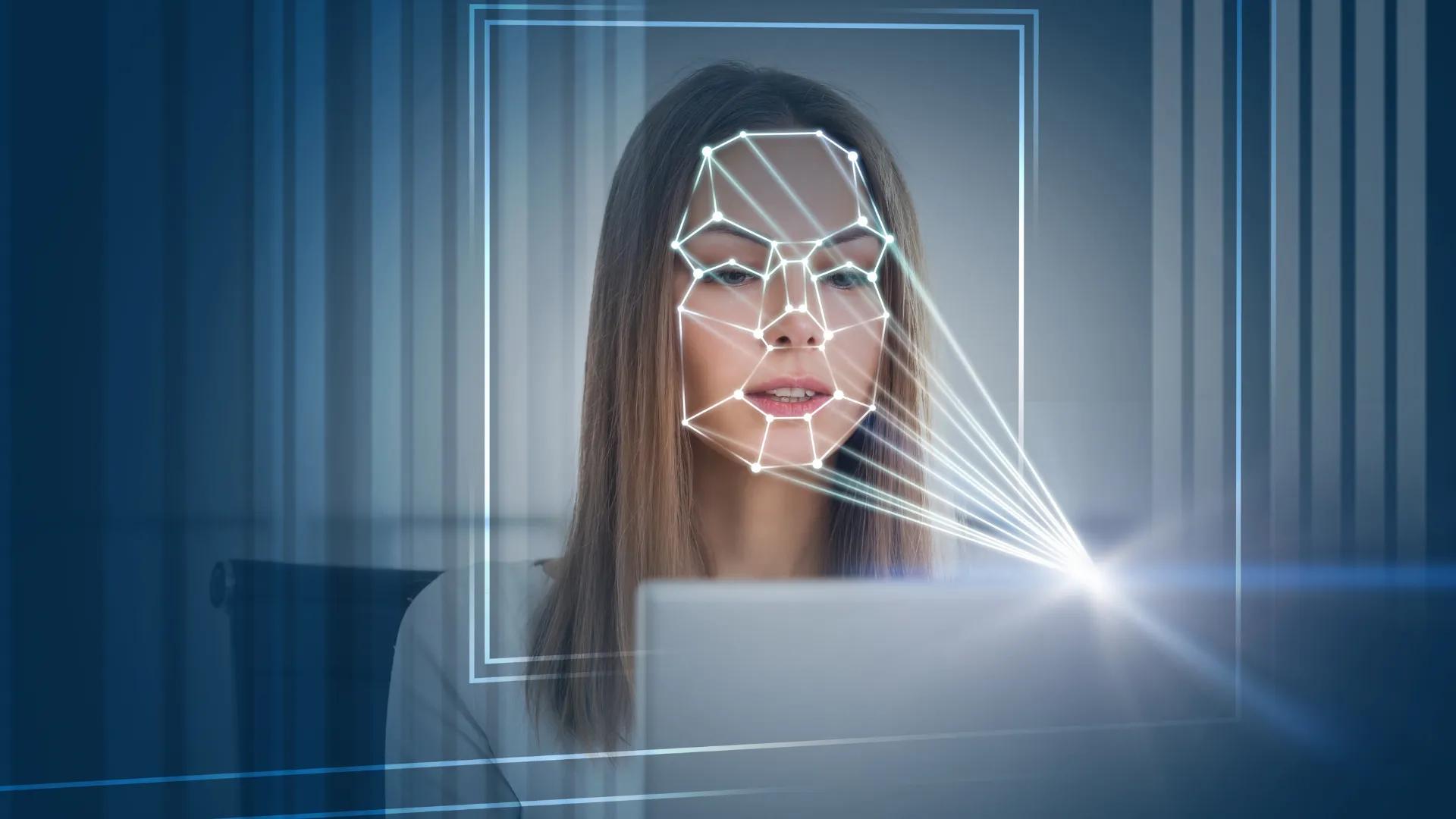
4. Real-world Examples of Bias in AI
Examine real-world examples and case studies of bias in AI, including hiring algorithms and facial recognition systems, and their impact on individuals and society.
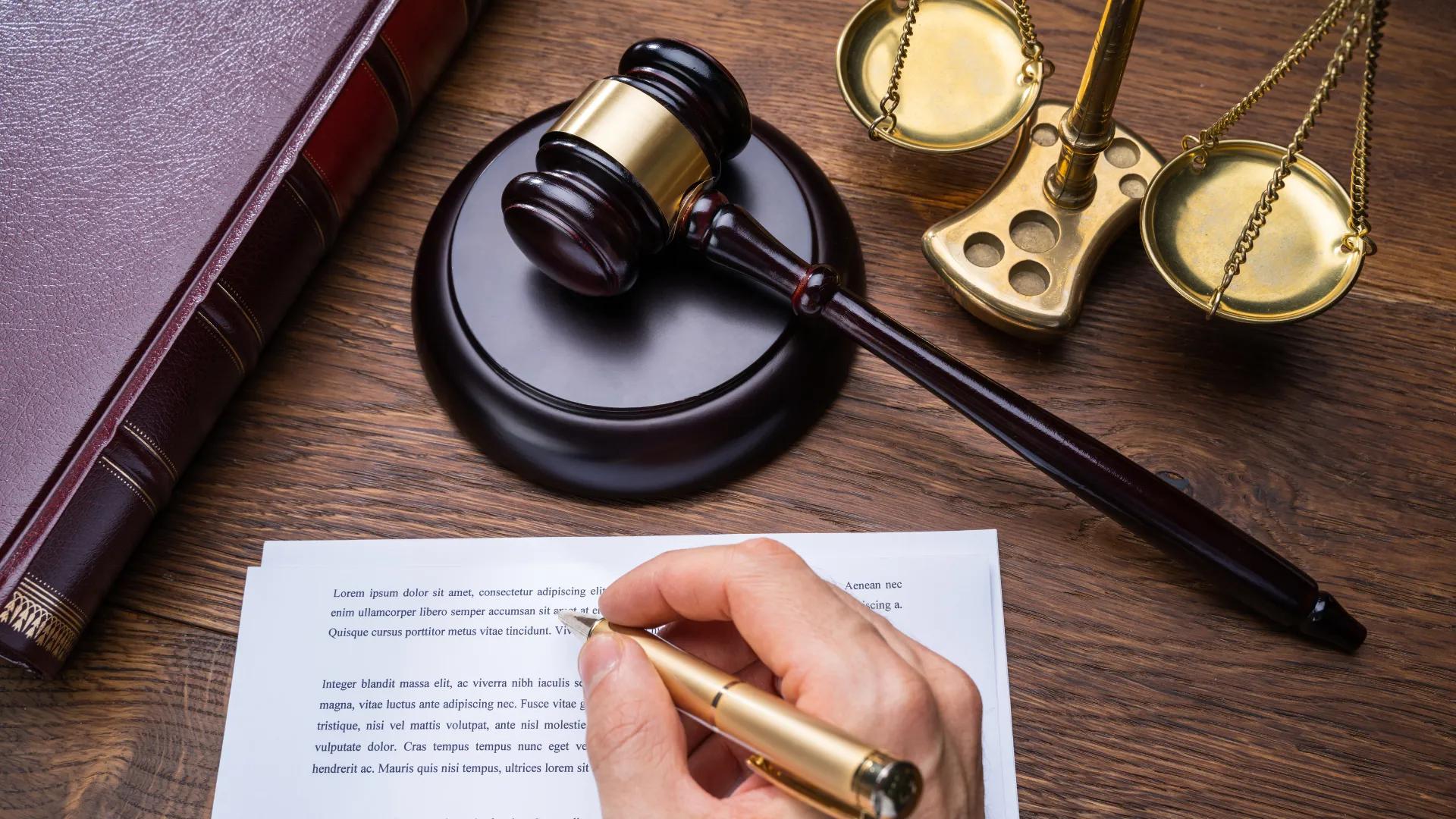
5. Societal Consequences
Discover the societal consequences of biased AI, including exacerbation of societal inequalities, legal risks, and reputational damage.
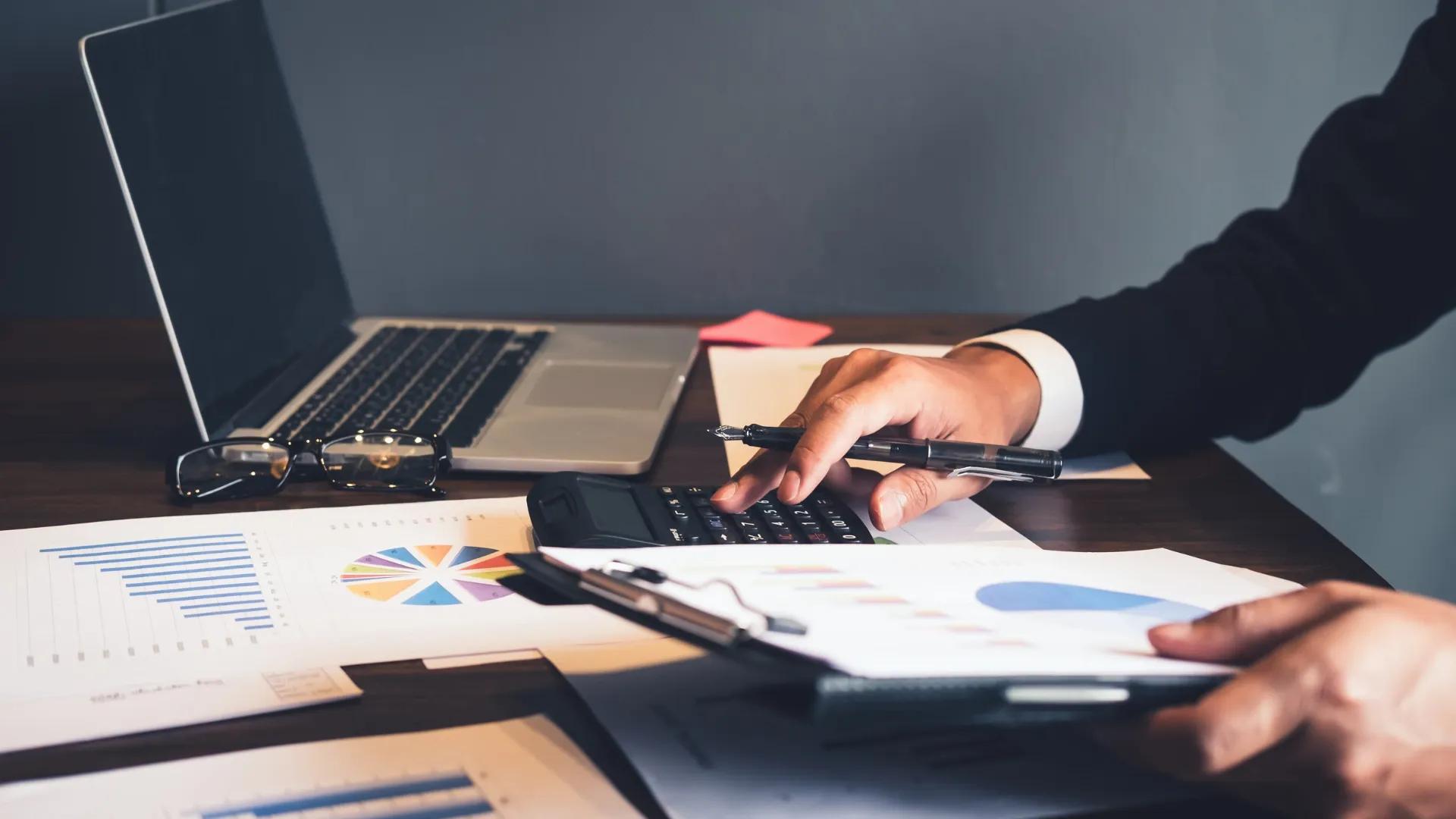
6. The Auditing Process
Learn about the systematic auditing process for AI systems, including defining objectives, data analysis for bias detection, and continuous monitoring.
%20(22).webp&w=3840&q=75)
7. Data Analysis for Bias Detection
Explore the steps of data collection, data processing, and data visualisation to detect biases in the model's data.
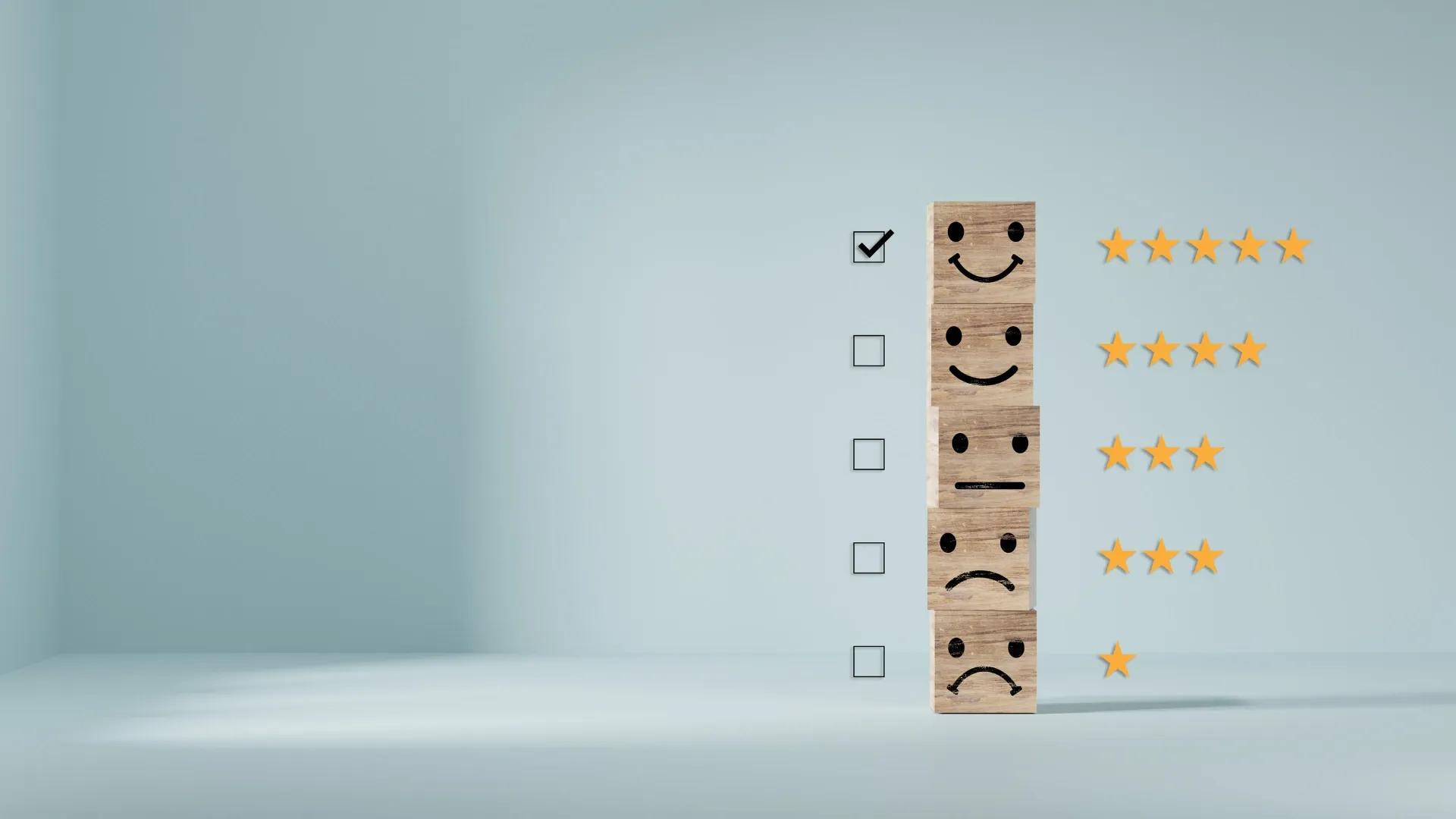
8. Model Evaluation Metrics for Bias
Understand various metrics for evaluating bias in AI models, including fairness metrics and confusion matrices.
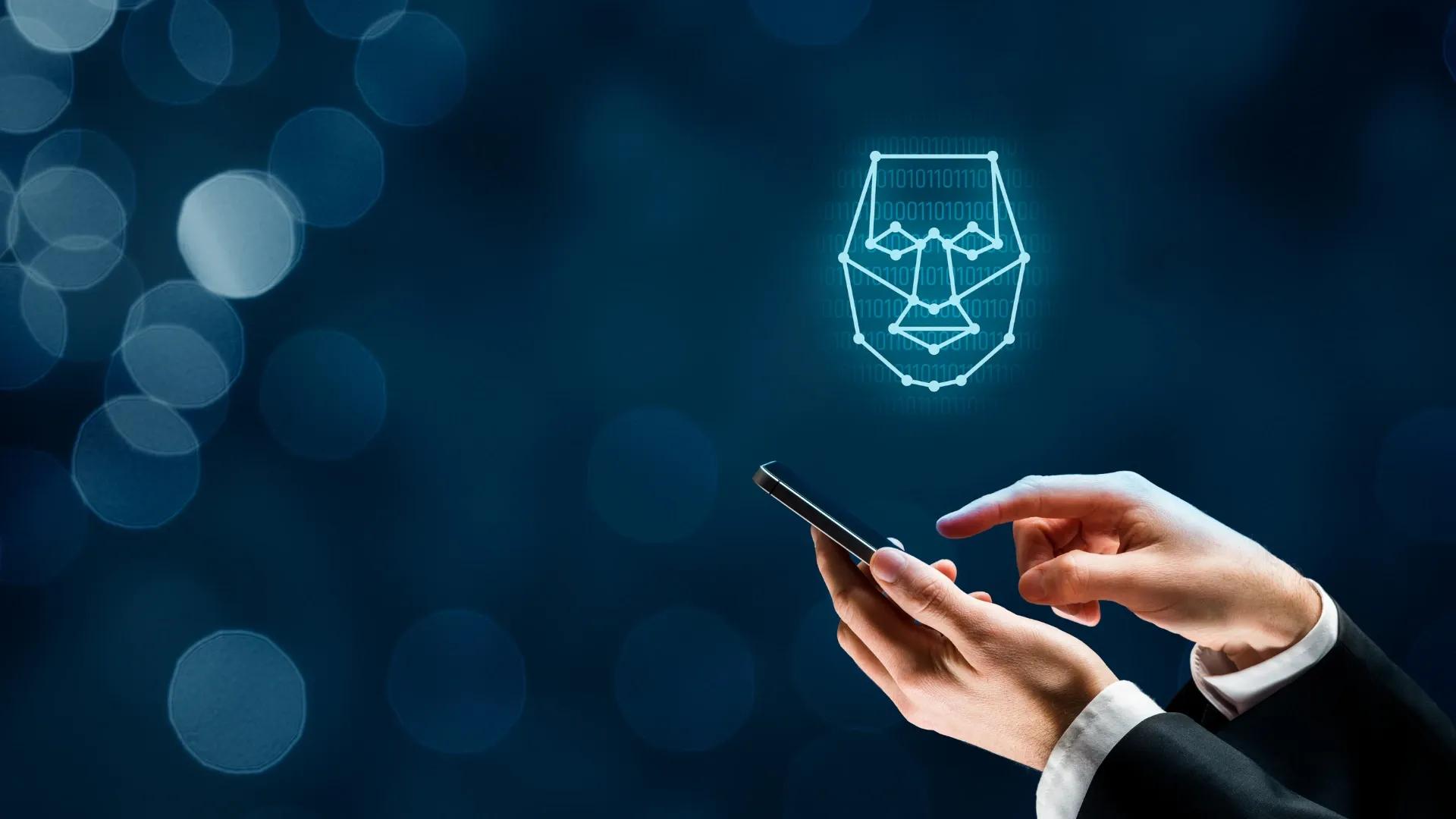
9. Bias Detection Techniques
Learn about pre-processing, in-processing, and post-processing methods for bias detection and mitigation in AI models.
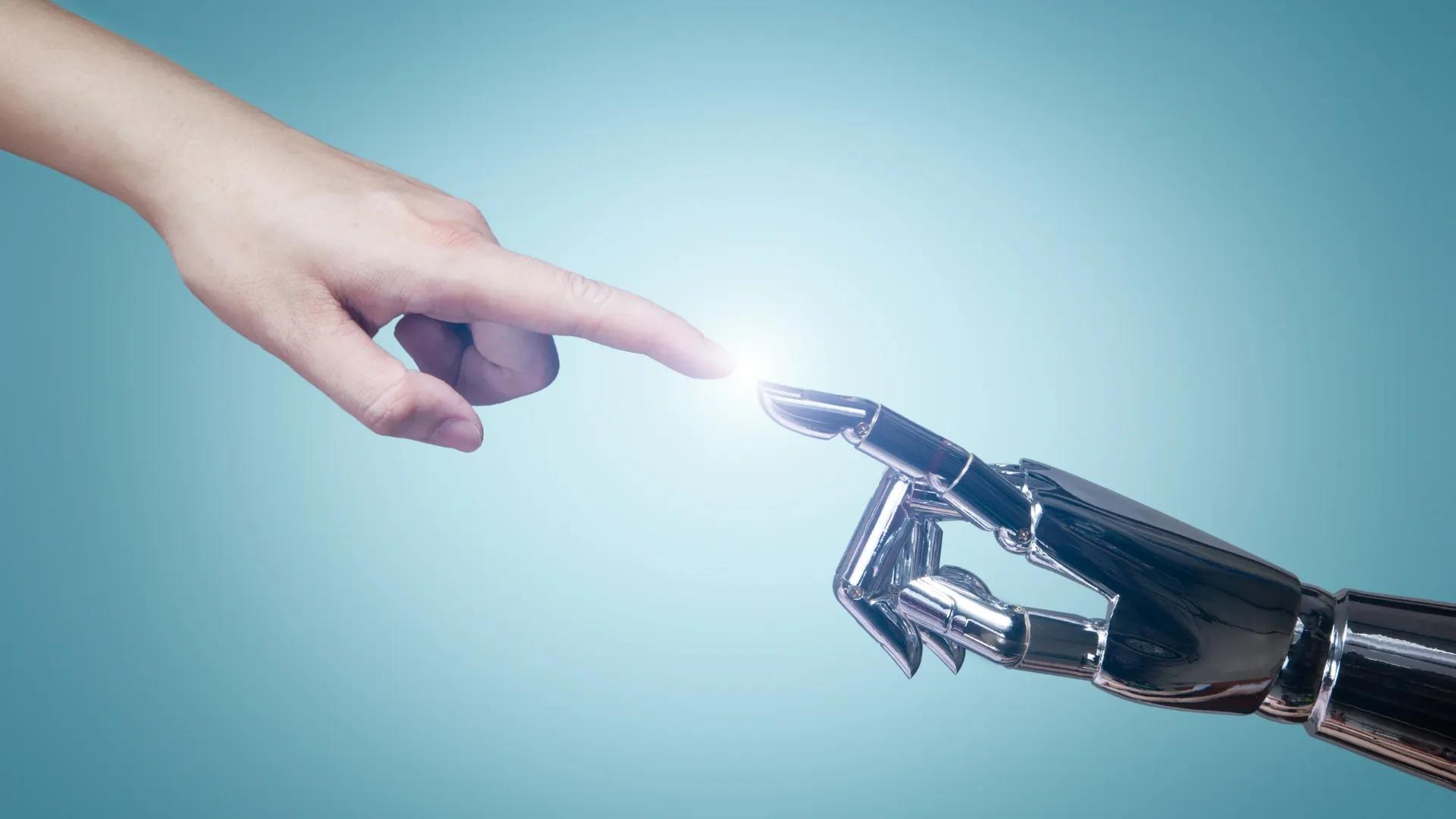
10. Interpretable Models and Explainable AI
Discover the importance of transparency in AI models and tools for explainable AI such as LIME, SHAP, and Counterfactual Explanations.
Ready to ensure your AI systems are fair, unbiased, and ethically sound? Download the full PDF guide now to gain detailed insights, practical examples, and a step-by-step approach to mastering the auditing of model bias in AI.
Don't miss out on the opportunity to create ethical and accurate AI systems with our comprehensive guide.
Download the Full Guide:
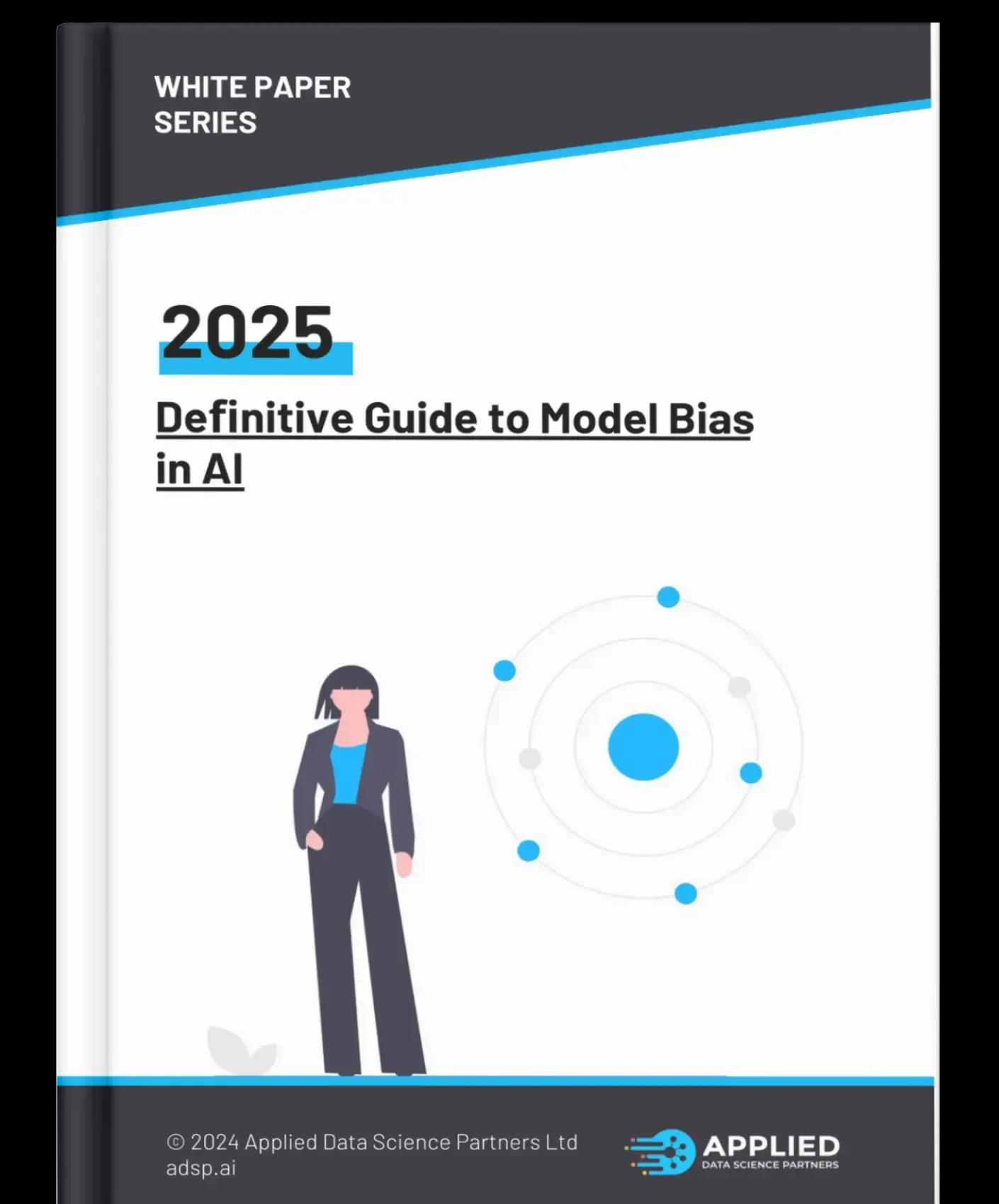